The world is changing rapidly. It’s no longer enough to just think about the present and the near future; businesses must now be prepared for what might happen in the future. While this may seem like an impossible task, it can be achieved with the help of machine learning and IoT devices.
An introduction to machine learning
Machine learning is one of the most exciting and powerful tools in the AI toolkit. It’s also a subset of artificial intelligence, which is itself an umbrella term that covers all technologies that aim to mimic human intelligence.
Machine learning services with Data Science UA refers to a method by which computers learn without being programmed or explicitly told how to do so. Instead, they’re given examples of data that have already been labeled as correct answers (or labels), and then use this information to “train” themselves on how best to perform their tasks, for example: recognizing objects in photos or videos; identifying voices from recorded conversations; processing text documents into natural language; making predictions about future events based on current observations…you get the idea!
How machine learning works with IoT devices
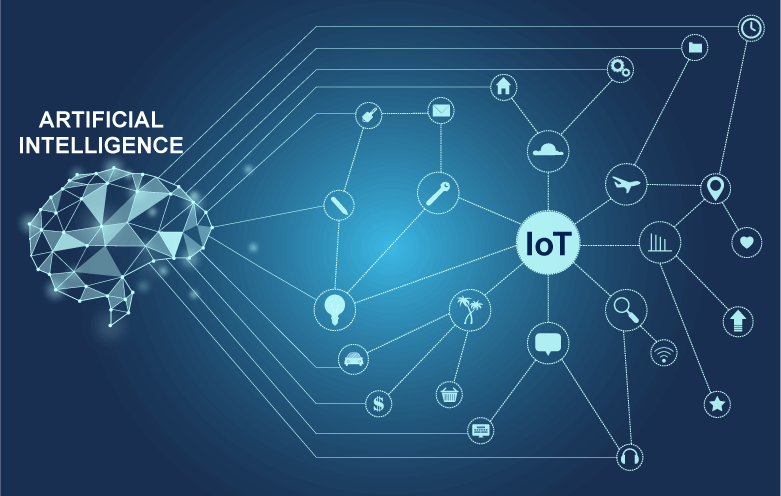
Machine Learning with IOT Devices
Machine learning is a set of algorithms that can learn from data. It’s used to make predictions, and decisions and improve the performance of IoT devices.
Machine learning can be applied to many different types of IoT devices including sensors that collect data about their environment or processes, analytics engines that process this information, and actuators that take action based on insights gained from machine learning algorithms.
- Improve performance: The goal here is for your machine learning model to predict what will happen next in order for you to intervene before any damage occurs (e.g., preventative maintenance). For example: If an engine sensor shows signs of overheating it may trigger an alert before overheating occurs so that someone can take action before damage occurs.
- Improve functionality: Machine learning models can be used as part of decision-making processes within various systems such as self-driving cars, which help determine road conditions ahead based on historical data.
- Make predictions: This allows companies like Amazon Echo or Google Home speakers to make recommendations based on past searches/shopping history etc.
Precisely accurate and efficient monitoring
Machine learning is a type of artificial intelligence that allows computers to learn without being explicitly programmed. It’s a very broad field, and there are many different types of machine learning algorithms (including deep learning and neural networks). Still, for this article, we’re going to focus on supervised learning.
Supervised learning uses labeled data sets to train an algorithm so that it can predict outcomes based on new information it has never seen before. An example would be if you had a dataset containing thousands of photos with labels such as “cat” or “dog” attached to them, you could use this information to build an algorithm capable of recognizing whether any given image contains either animal in its future predictions.
Efficient prediction based on history and past events
Machine learning is used to predict future events based on history and past events. This can predict when a machine will break down, when it needs servicing, or when it will need tuning.
- Machine learning for predictive maintenance: A company may use machine learning to detect when a piece of equipment is likely to fail so that they can schedule repairs in advance.
- Machine learning for predictive scheduling: A company could use this kind of technology to determine which orders should be processed first based on historical data about customer demand or other factors that affect shipping times such as weather conditions (i.e., shipping by air versus by sea).
Conclusion
In conclusion, integrating machine learning with IoT with AI by Data Science UA has many benefits. It allows for more precise and efficient monitoring, specific prediction based on history and past events, better decision-making based on real-time data from multiple sources, and more accurate forecasting models to predict future outcomes. In addition, this technology has applications in many industries such as healthcare where doctors can use it for better diagnosis, or financial services where banks could use it for fraud detection purposes. Read more from Wikipedia