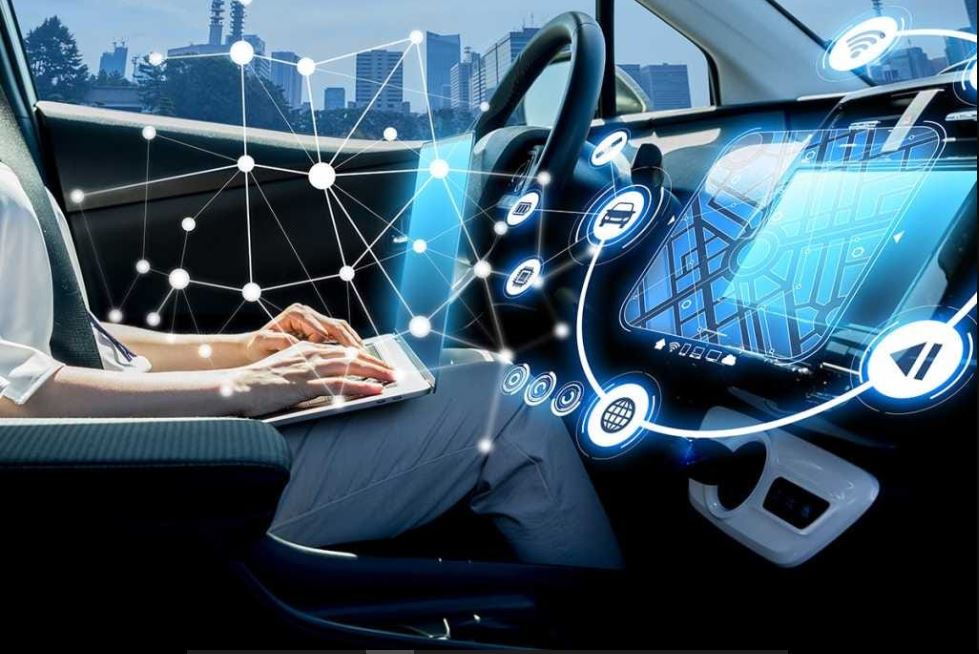
Introduction
Have you ever imagined a world where cars drive themselves? It’s not just science fiction anymore, as self-driving cars are on the rise, and they’re set to completely transform how we get around. Get ready to buckle up as we dive into the incredible journey of these autonomous vehicles. And guess what? We’ve got a game-changer in the mix – Mindy Support Data Annotation. They’re like the unsung heroes behind the scenes, helping teach self-driving cars how to navigate our streets. But that’s not all – we’re also going to unravel the mystery of video labeling, a crucial piece of the self-driving puzzle you won’t want to miss.
Evolution of Self-Driven Cars
The journey towards autonomous vehicles has been marked by remarkable milestones, each pushing the boundaries of what was once thought possible. In 1987, Ernst Dickmanns unveiled a self-driving car that autonomously maneuvered through traffic-free environments, challenging the conventional limits of machine capabilities. Fast-forward to the early 2000s and the DARPA Grand Challenge, where self-driving vehicles conquered complex desert terrains, showcasing advancements in sensing and decision-making.
The Crucial Role of Data Annotation and Video Labeling:
Now, let’s delve into the heart of the matter: the role of data annotation, particularly the transformative concept of video labeling. Data annotation serves as the cornerstone for training self-driving car algorithms. It’s here that Mindy Support Data Annotation comes into play, meticulously creating labeled datasets that power the learning capabilities of autonomous vehicles. And what is video labeling? This is where the magic unfolds. Video labeling involves annotating various elements within video footage – from identifying objects and pedestrians to discerning road signs and lane markings. It’s akin to teaching the vehicle to decipher its visual surroundings, an essential skill for making informed decisions on the road.
AI and Machine Learning’s Vital Role:
Fueling the astonishing capabilities of self-driving cars is the fusion of AI and machine learning. These technologies serve as the bedrock upon which autonomous vehicles rest. By harnessing the power of AI and machine learning, vehicles process copious amounts of data collected from sensors, cameras, and LIDAR systems. This real-time analysis of data – encompassing road conditions, traffic dynamics, and potential obstacles – is facilitated by intricate algorithms. Over time, these algorithms learn and adapt, guiding the vehicle’s actions based on informed decisions. The significance of AI and machine learning is monumental; they are the cognitive forces that bridge the gap between self-driving concepts and tangible on-road realities.
From Speech Recognition to Autonomous Intelligence:
The impact of AI and machine learning stretches beyond autonomous navigation. Take, for instance, Windows’ groundbreaking introduction of speech recognition software in 1997. This technology now seamlessly integrates with modern navigation systems, including Google Maps. The result? A revolution in the driving experience. By reducing the need for visual interaction with screens, speech recognition elevates safety by minimizing distractions. This evolution showcases technology’s ability to address autonomy, user interface, and safety concerns.
Learning from the Past, Driving into the Future:
Yet, these monumental strides have not been without their challenges. The beauty of AI and machine learning lies in their capacity to learn from past experiences. Machine learning algorithms continuously absorb data, enabling them to analyze and evolve based on historical patterns. This learning process ensures that self-driving cars refine their decision-making capabilities over time, enhancing their proficiency and safety.
Conclusion
As we witness the evolution of autonomous vehicles, it’s evident that AI and machine learning serve as the bedrock of this transformative journey. These technologies empower self-driving cars to perceive, comprehend, and react to intricate road scenarios, charting a course toward a safer and more efficient future of transportation.